The advent of computer vision - deep learning models trained on image data to classify, annotate, or otherwise characterize new images - offers a powerful toolkit for analyzing medical images. Here we highlight three creative ways computer vision is entering the medical sphere, and the pertinent questions facing this rapidly-moving field.
1. Medical Image Interpretation
Computer vision can augment or replace traditional medical image analysis techniques, and it truly shines at tasks that are "boring" and/or "impossible". A “boring” task, for example, would be searching a slide of breast tissue for malformed cells, while an “impossible” one would be consistently and accurately quantifying the percentage of tissue exhibiting a particular feature in such a slide. As Stephane and Seth have written in previous DeciBio blog posts, the initiatives most likely to be successful are those that complement specific shortcomings in human interpretation - the “boring” and the “impossible” - rather than replace doctors entirely. PathAI, DeepBio, and Owkin, leaders in computational pathology, have demonstrated success at automatically annotating slide images and even combining image data with other datasets. Meanwhile, companies such as Lunit, InformAI, and CureMetrix provide radiology image interpretation support tools for radiology; recent weeks have sparked debate over using machine learning to identify COVID-19 cases from chest X-rays.This paradigm of human-machine collaboration avoids some of the typical concerns about algorithms holding decision-making power, but the field has not been immune from public scrutiny even outside times of crisis. Training these algorithms requires massive quantities of patient data, and that data often needs to be accurately (and expensively) annotated. Paige, another digital pathology machine learning company, ignited outcry in 2018 over its deal with Memorial Sloan Kettering granting Paige exclusive access to certain MSK repositories of patient slides. Going forward, stakeholders need to establish and follow best practices for the use and potential profit from patient data.
2. Facial Analysis
Deep learning techniques are not only giving the medical community new ways to analyze medical images - they are expanding the definition of what a medical image is. One example is FDNA, a company offering a phenotype-to-genotype syndrome-matching tool. Using a standard facial portrait of a patient, their software uses a similar-image-search approach to identify possible genetic variants and syndromes to aid clinicians in differential diagnosis of rare dysmorphologies. Dthera Sciences, meanwhile, has developed a digital therapeutic that uses facial expression analysis to customize its content while treating patients with Alzheimer’s.These technologies and others that use facial images offer exciting opportunities for patients with unidentified syndromes, patients requiring resource-intensive therapy, and more. At the same time, they turn facial images into potential troves of interpretable health data. In a world where health information is (rightly) protected, while face portraits abound online, a technology that can extract the former from the latter breaks down the distinctions between data types and raises new concerns about privacy and medical confidentiality. It is up to the medical and ethical communities to assess the clinical benefits alongside possible privacy concerns as technologies offer novel ways to interpret ubiquitous, traditionally non-medical information.
3. Encoded Data Visualization
Lastly, computer vision experts are turning their attention to problems that don’t inherently contain image data at all. DeepVariant, Google’s project to harness their image analysis expertise for variant calling, is one example that has reported high-quality results. DeepVariant encodes read bases, quality scores, and other read features into RGB pixels to generate images of read data, then feeds the image into a convolutional neural network (CNN), which can output likelihoods for different genotypes. It’s the old adage of “when you have a hammer, everything looks like a nail”, but generating surprisingly accurate results; in 2018 DeepVariant outperformed existing state-of-the-art techniques and has achieved gains in accuracy since. Another company, AtomWise, uses the power of CNNs for drug discovery, encoding possible atoms as pixels and using computer vision for target identification.With few exceptions, nearly every deep learning model to date faces the same challenge for clinical use: their nature as black-box algorithms prevents detailed analysis of how different features lead to the output. This can, in some sense, shut humans out of the analysis process. It can be nearly impossible for humans to sense-check an algorithm’s output or compare its methodology to existing processes without a traceable decision-making process or meaningful features. The advantages of human-machine partnership demonstrated via computer-assisted pathology discussed above, for example, are lost in these end-to-end black-blox algorithms.Another general challenge beyond algorithmic interpretability is the perennial issue of large vulnerable datasets. The proliferation of digital medical images has not guaranteed secured storage; a TechCrunch report earlier this year found that over a billion patient medical images are improperly secured. In order to generate useful insights, these algorithms require large training sets, and once trained, their usefulness encourages further digitization. As clinical algorithms drive demand for digitized images, data security must not fall out of focus.These developments and challenges illustrate just a few of the many different ways that advancements in computer vision technology are benefitting the medical sector, and the pressing questions they raise as the medical computer vision landscape develops. Together, they show the diversity of data for which computer vision can aid in interpretation, as well as some of the open questions that remain to be addressed as the technology progresses.
Julia daniel
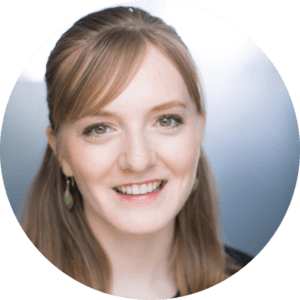
Julia is a Senior Analyst at DeciBio with a focus on health technology, clinical diagnostics, and the impact of machine learning on precision medicine. Julia’s work at DeciBio includes market intelligence, voice-of-customer research, and data tools development. Connect with her on LinkedIn or reach out at daniel@decibio.com.Disclaimer: Companies listed above may be DeciBio clients and/or customers